To watch the webinar that is the basis for this article, visit the HIMSS Resource Hub.
In the ever-evolving landscape of healthcare, Artificial Intelligence (AI) is emerging as a transformative force. A recent HIMSS Webinar, presented by Jeff Fuller, VP of Delivery – Analytics, and Carole Piovesan, Co-Founder and Partner at INQ Consulting, delved into the multifaceted impact of AI on healthcare. The session – recorded and accessible here – provided a deep dive into strategies for governance, culture, and ethics, aiming to enhance AI adoption in healthcare, understand AI governance frameworks, manage organizational change for AI integration, and build public trust in AI. Specifically, the webinar and this summary aim to:
- Review methods to enhance AI adoption in healthcare
- Understand AI governance frameworks
- Manage organizational change for AI integration
- Build public trust in AI
AI Benefits & Use Cases
Benefits of AI in Healthcare
The benefits of AI in healthcare are vast and varied. From better patient outcomes and system efficiency to administrative and clinical applications, AI is poised to revolutionize the industry. Key areas where AI can make a significant impact include:
- Better Patient Outcomes: AI can help improve patient care by providing more accurate detection and diagnoses, personalized treatment plans, and accelerated research and development.
- System Efficiency: AI can streamline administrative processes, reducing waste and improving overall efficiency. It can automate routine tasks, freeing up healthcare professionals to focus on more critical aspects of patient care.
- Less Waste: AI can help identify and eliminate inefficiencies in the healthcare system, including:
- Failure of care delivery / poor adherence to clinical best practices
- Failure to coordinate care
- Overtreatment, including the provision of low-value care
- Unreasonably high prices
- Administrative complexity
Common Use Cases & Scenarios
The webinar also explored several common use cases and scenarios for AI in healthcare:
- Customer Service Chatbots: AI-powered chatbots can provide patients with instant answers to their questions, improving customer service and satisfaction.
- Intelligent Patient Engagement: Help engage patients more effectively, providing personalized care and support.
- Coding & Documentation Process Automation: Automate the coding and documentation process, reducing errors and saving time.
- Personalized Care Management: Help manage patient care more effectively, providing personalized treatment plans and monitoring patient progress.
- Predictive Rising Risk Segmentation: Identify patients at risk of developing certain conditions, allowing for early intervention.
- Early Disease/Diagnosis Surveillance: Help detect diseases early, leading to better outcomes.
- Capacity Planning/Transitions: Assist in planning and managing patient transitions, ensuring a smooth and efficient process.
- Genetic Sequencing: Analyze genetic data, providing insights into a patient’s health and potential risks.
Challenges with AI
Despite its many benefits, AI also presents several challenges. There are several key areas of concern:
- Public Trust: Ensuring the public and impacted stakeholders are on board and willing to interact with or use AI systems
- Oversight & Accountability: Determining when to involve humans and who should be accountable for AI system outputs.
- Robustness & Cybersecurity: AI systems should fulfil their intended purpose, produce consistent results, and be resistant to adversarial attacks.
- Transparency & Explainability: Determining what to communicate externally and to impacted stakeholder groups
- Bias & AI Risk Mitigation: AI systems pose novel risks such as bias due to their black-box nature.
AI Adoption
Why Data Governance for AI
Data is a foundational component of almost everythingwe do in healthcare today. If the foundation is not of high quality and well understood, success is negatively impacted. Data regulations continue to expand in both volume and complexity. To minimize risk and bias, and to maximize reliability transparency into how data flows through the organization is critical. Data-driven decision making enables us to learn from the past, gain a deep understanding of current state, and to select the best paths forward for augmenting workflows with AI. Machine Learning and Artificial Intelligence depend on well-defined and high-quality data as a requirement for effective modelling.
Critical Elements of AI Adoption
Several elements are critical for successful AI adoption:
- Data
- Modernize data assets for reusability in AI applications
- Identify data silos & redundancies
- Build a community around data expertise
- Prioritize data governance, data quality, & data stewardship
- Governance
- Defined & aligned organizational success criteria
- Effective cross functional governance and delivery
- Rapid decision making with clear escalation path
- Subject Matter Expert involvement & empowerment
- An ineffective governance is the same as no governance
- Change Management
- Embrace the benefits of AI: end user agency, iterative accuracy & effectiveness, augmented workflows, integrated learning & communications
- A combination of readiness, engagement & adoption activities
- Human interaction is one of the things healthcare does right, AI should be human-first
AI Governance Frameworks
What is AI Governance?
AI governance involves structures, processes, and tools designed to provide answers to common AI-related questions. It emphasizes the importance of having a robust governance framework to ensure that AI systems are used responsibly and effectively. Some of the questions include:
- Who has the decision rights and accountabilities for the data?
- How do we maintain compliance with changing best practices?
- How do we mitigate biases to ensure fair outcomes?
- What is the role of diverse stakeholders?
- How do we balance innovation with ethical oversight?
Typical Challenges in Governance
There are several common challenges in AI governance. These challenges include siloed knowledge and responsibilities, competing priorities, and a lack of engagement:
- Siloed Knowledge & Responsibilities: issues with inconsistent knowledge, redundancies, and inefficiencies
- Competing Priorities: organizational politics, strategic/tactical conflicts, high demand for insights with limited analytic resources, and a lack of enterprise portfolio rationalization
- Lack of Engagement: no self-service enablement, inconsistent operating models, unclear job roles, and mixed sentiments ranging from excitement to fear
AI Governance Builds on Your Existing Capabilities
AI governance should leverage existing capabilities within an organization. Several key areas are foundational to effective AI governance, across four main categories:
- Data
- Data Governance / Quality
- Data Privacy
- Data Sourcing and Consent
- Data Access
- Model
- System Validation & Robustness
- Transparency
- Model Bias & Fairness
- Model Security
- Use & Output
- Notice & Disclosure
- Ongoing Monitoring & Evaluation
- Impact Assessment
- User Education
- Ethics
- Ethical Principles &Guidelines
- Stakeholder Engagement
- Responsible Innovation
Key AI Governance & Risk Management Artifacts
There are several key artifacts essential for AI governance and risk management. These include:
- AI Policy
- AI Risk Committee
- AI Risk Assessment
- AI Vendor Assessment
- AI Training & Education
- Ongoing Monitoring & Reporting Procedures
- Notice & Disclosure for AI
- Technical Testing of AI Systems
Managing Change
Readiness for AI Adoption
Workforce and organizational readiness are essential for AI adoption:
- Workforce Readiness:
- Who are the humans that will interact with the AI, and how will they interact?
- How can you prepare them for change?
- Who will oversee the system over time?
- Do we have the talent needed to execute?
- Organizational Readiness
- What outcomes are we trying to impact?
- What workflows will change?
- How much effort is required to implement the solution?
- What organizational governance do you need in place to address legal & ethical challenges?
Get Ready
There is a lot to be excited about. AI is poised to have the most transformative impact in the history of medicine. Everyone should get ready — there is a cost to not doing anything today. Healthcare IT leaders must proceed with a comprehensive approach that considers organizational, cultural, and strategic readiness. Gain clinical, business, and technical stakeholders’ trust and collaboration as early as possible, and don’t overlook the data, governance, and change management work needed to maximize success.
Ready to learn more?
Discover AI and data governance solutions that meet your organization’s needs.
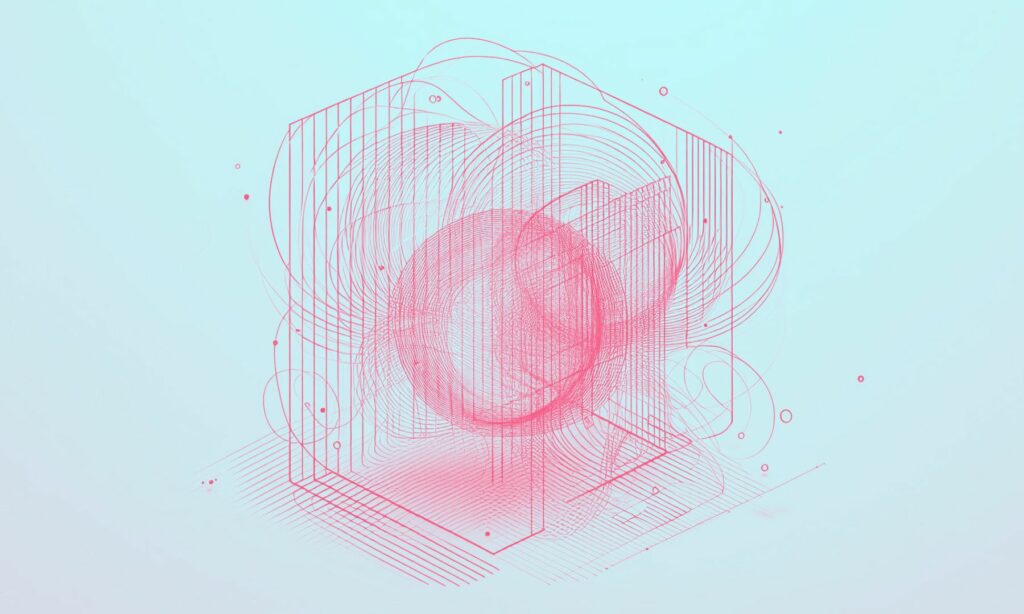
About the Webinar Participants
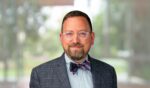
Jeff Fuller | Vice President, Delivery | Divurgent
Jeff has spent his 27-year career as a health leader and collaborator, driven by a passion for innovating on care strategies that emphasizes a whole person view of high value care. As a subject matter expert in analytics, clinical transformation, and strategy, Jeff has supported meaningful partnerships and collaborations to achieve innovation and improvement evidence through real measurable results.
Prior to joining Divurgent to lead the Analytics practice, Jeff led product strategy at a patient engagement solution provider and prior to that led the startup of the enterprise analytics team at UNC Health as executive director, where he led a team of data scientist to the leading edge of the AI evolution in the healthcare industry. Jeff has also led analytics at Atrium Health and MUSC Health. Jeff is Board Certified as a Fellow of the American College of Healthcare Executives and holds a Bachelor of Health Administration and a Master of Science in Management. To learn more about Jeff, visit his LinkedIn.
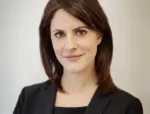
Carole Piovesan | Co-Founder and Partner | INQ Consulting
Carole is co-founder and principal at INQ Consulting, focusing her practice on privacy, cyber readiness, data governance and artificial intelligence (AI) risk management. She regularly advises clients on a wide range of matters related to privacy, cyber readiness and breach response, data governance, ethical AI and responsible innovation.
She is a member of theOECD.AI Policy Observatory, and has contributed to numerous organizations including in the Global Partnership on AI, the Global Task Force to Promote Trusted Sharing of Data at the German Marshall Fund of the United States, the Standards Council of Canada’s Data Governance Collaborative, and the National Advisory Council on AI in Healthcare.
Carole regularly teaches, speaks and writes on topics related to cybersecurity, privacy, data law and AI. She has been featured on the CBC, the Globe and Mail, The Logic, and Betakit. She has testified beforeCanada’s House of Commons Standing Committee on Access to Information, Privacy and Ethics. Carole is also an adjunct professor at the University of Toronto, Faculty of Law where she lectures on AI regulation.